Researchers Test AI for Hawaiian Language Transcription
Tests of Hawaiian speech recognition had a 22% error rate, despite never having been trained on labeled audio data in the language.
In a new study, researchers have demonstrated that large artificial intelligence models can significantly improve automatic speech recognition (ASR) for the Hawaiian language by leveraging existing text data. The findings, published Wednesday, provide hope for using AI to aid language preservation and revitalization efforts for under-resourced and endangered languages like Hawaiian.
The research team, led by Kaavya Chaparala and Guido Zarrella of The MITRE Corporation, evaluated the performance of Whisper, an AI model developed by OpenAI, on transcribing spoken Hawaiian to text. Out of the box, the largest Whisper models were able to transcribe Hawaiian audio with a word error rate of about 22%, despite never having been trained on labeled Hawaiian audio data.
“We found the largest Whisper models could transcribe Hawaiian audio with word error rates of about 22%,” said Chaparala, the lead author of the study. “This zero-shot learning capability, where the AI can perform the task without seeing any Hawaiian training data, is very promising.”
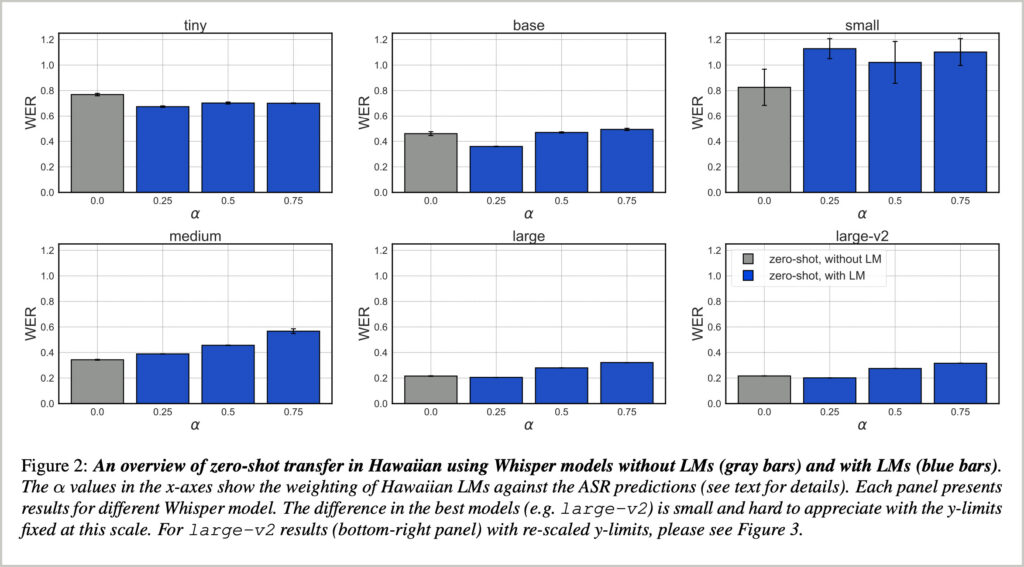
To further improve performance, the researchers incorporated an external Hawaiian language model into Whisper. The language model was trained on a corpus of about 1.5 million words of Hawaiian text. By combining the language model with Whisper, the researchers reduced the word error rate to about 20% — a small but statistically significant improvement.
“By incorporating a Hawaiian language model, the best Whisper model achieved a word error rate of about 20%,” explained Zarrella, a co-author. “While the 1-2% absolute improvement may seem small, for low-resource languages like Hawaiian which could use better automatic speech recognition to accelerate preservation efforts, any significant improvement in performance is welcome.”
Hawaiian, an endangered language spoken by less than 0.1% of Hawaiians, faces challenges such as a lack of labeled training data that have hindered the development of high-quality ASR systems. But as this study demonstrates, leveraging AI and making use of available text data can help bridge that gap.
“There are dozens of hours of labeled Hawaiian audio but millions of pages of Hawaiian text available right now,” said co-author Bruce Torres Fischer of the University of Hawai’i at Hilo. “By showing how we can use that text data to meaningfully improve AI speech recognition in Hawaiian, this work makes an important contribution to our language revitalization efforts.”
The researchers said their approach of combining an external language model with an AI foundation model like Whisper could potentially be applied to aid automatic speech recognition in other low-resource languages as well.
“What can other low-resource language communities learn from this study? We conjecture that many languages are like Hawaiian in having much less labeled data than unlabeled data like text corpora,” said co-author Oiwi Parker Jones of the University of Oxford. “Our results show it is worth trying to use all the data you have to improve AI speech recognition performance.”
However, they cautioned that Hawaiian may not be fully representative in terms of its characteristics and the data available. More work is needed to evaluate how well the approach generalizes to other low-resource languages.
Analysis of the AI model’s errors also suggested areas for future improvement. For example, the model struggled with distinguishing short and long vowels in Hawaiian, as well as glottal stops. The authors hypothesized that fine-tuning the model on labeled Hawaiian audio data could help correct these errors.
“Ultimately, how well our approach works for other low-resource languages is an empirical question,” said Jones. “But for languages that have few options, we offer hope: it is worth trying, as Hawaiian tradition teaches, to use all the data you have.”
The researchers plan to continue improving the AI models for Hawaiian ASR by training on more text data and pseudo-labeling unlabeled Hawaiian audio. They are working with the Hawaiian community to gather larger amounts of labeled data as well.
“Advances in automatic speech recognition have predominantly benefited a few global languages. To date, this has left speakers of low-resource languages, such as Hawaiian, at a disadvantage,” said co-author Larry Kimura of the University of Hawai’i at Hilo. “High-quality Hawaiian ASR developed through studies like this would support our language preservation and revitalization efforts.”
The study was funded by the Medical Research Council, Royal Society, National Science Foundation, National Endowment for the Humanities, and Social Sciences and Humanities Research Council. It demonstrates the potential for AI to play a meaningful role in preserving endangered languages and knowledge for future generations.